
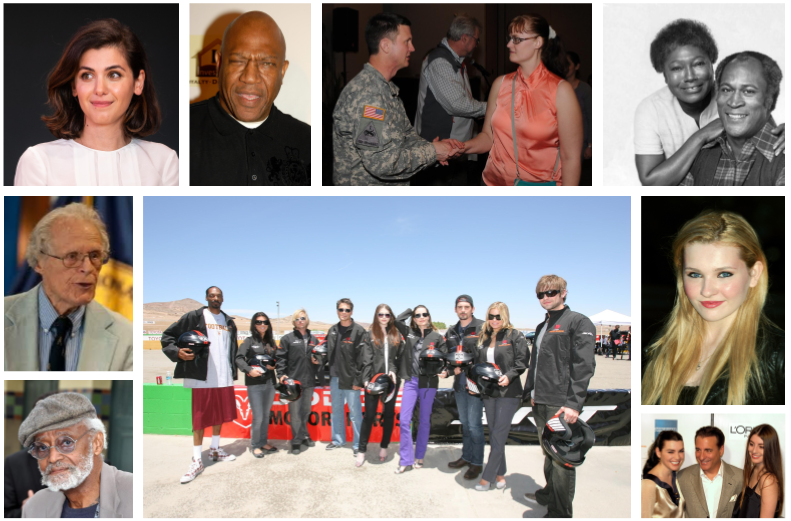
Fair Face Localization With Attributes (F2LA) Database
The presence of bias in deep models leads to unfair outcomes for certain demographic subgroups. In this work, we explore possible bias in the domain of \textit{facial region localization}. Being essential for all face detection and recognition pipelines, it is imperative to analyze the presence of such bias in popular deep models. Since most existing face detection datasets lack suitable annotation for such analysis, we web-curate the Fair Face Localization with Attributes (F2LA) dataset and manually annotate more than 10 attributes per face, including facial localization information. We design an experimental setup to study the performance of four pre-trained face detectors utilizing the extensive annotations from F2LA. We observe a high disparity in detection accuracies across gender and skin-tone and draw detailed analysis for observed discrepancies. We further discuss the role of confounding factors beyond demography in face detection.
F2LA database (CRC32: 175b48c3, MD5: 5a26955b053228135ac3a3f19e87c86e)
License Agreement + Citation
To obtain the password for the compressed file, email the duly filled license agreement to databases@iab-rubric.org with the subject line "License agreement for F2LA”. NOTE: The license agreement has to be signed by someone having the legal authority to sign on behalf of the institute, such as the head of the institution or registrar. If a license agreement is signed by someone else, it will not be processed further.
This database is available only for research and educational purpose and not for any commercial use. If you use the database in any publications or reports, you must refer to the following paper:
S. Mittal, K. Thakral, P. Majumdar, R. Singh, and M. Vatsa, Are Face Detection Models Biased?, IEEE Automatic Face and Gesture Recognition (FG), 2023.